Great speaker line-up at the ESSB conference! Register and submit abstracts for short talks and posters until August 31st!
Session 1 on December 12th: @joalunatkthse and Nicole Strittmatter
#spatialbiology #spatialtranscriptomics #spatialmetabolomics #ESSB
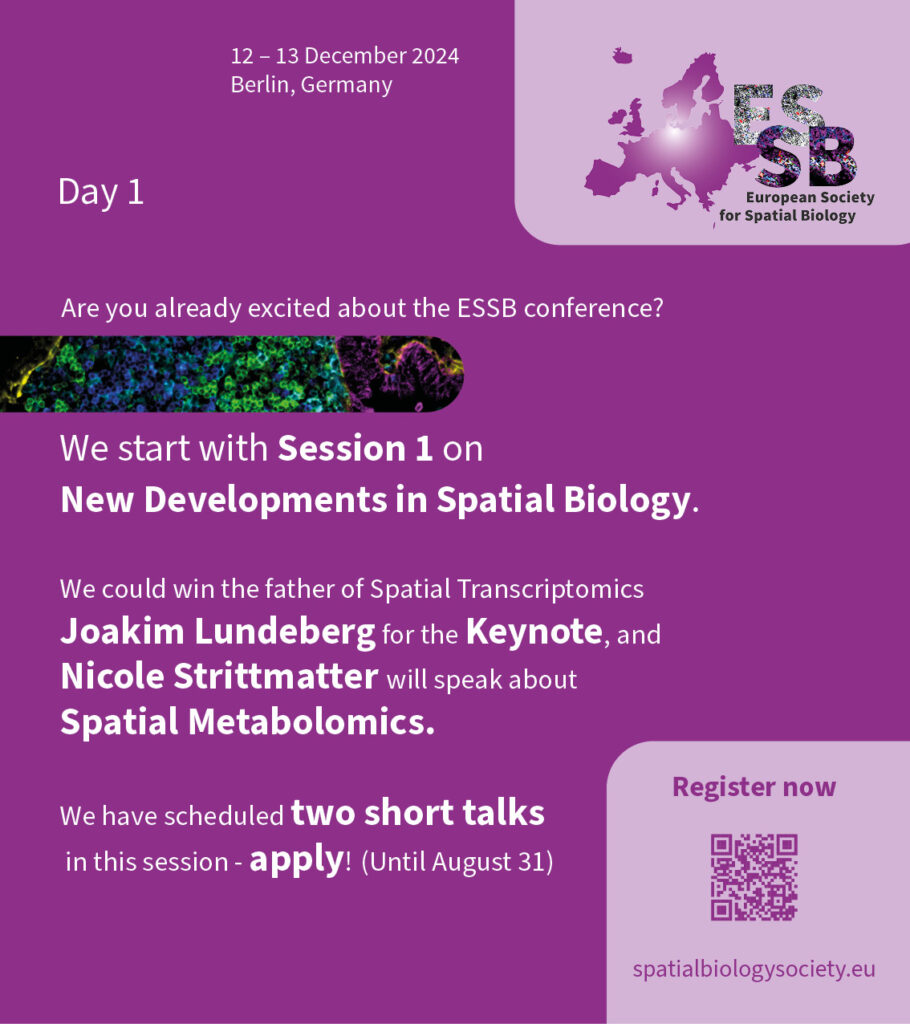